Recently, Elon Musk claimed that the supply of human-generated data used to train AI models, such as ChatGPT, is nearing depletion. Although he didn’t provide evidence, other tech leaders and earlier studies have suggested the same, estimating that genuine human-generated data could run out within the next two to eight years.
This looming shortage stems from the inability of humans to produce data—text, images, and videos—at a pace that matches the vast and growing demands of AI models. If this prediction holds true, developers and users could face significant challenges as tech companies increasingly turn to synthetic data as an alternative.
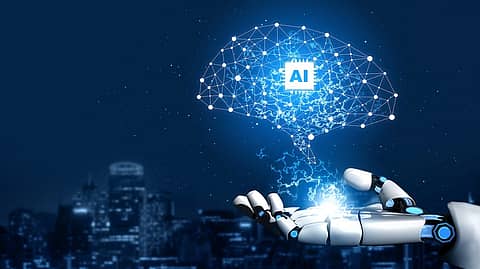
The Role of Real Data in AI Development
Real data, created by humans, forms the foundation of AI training. It encompasses content collected from surveys, experiments, websites, and social media. Valued for its authenticity and ability to capture a wide array of contexts, real data helps AI systems perform tasks with accuracy and reliability.
However, real data is far from perfect. It often contains biases, errors, and inconsistencies, which can result in flawed AI outputs. Preparing real data for AI training is also time-intensive, with up to 80% of development time spent on collection, cleaning, labeling, and validation processes.
The growing scarcity of real data underscores the need for alternative approaches, as human efforts alone cannot keep up with AI’s data demands.
>>>H013106 Replacement Battery for iFlytek T10 T20 CTGPRO1000A
Synthetic Data: A Promising Alternative
Synthetic data is artificially generated by algorithms, like the text produced by ChatGPT or images from DALL-E. It offers a potential solution to the data shortage by being faster and more cost-effective to produce. Unlike real data, synthetic data is unlimited and can be designed to address specific ethical or privacy concerns, such as protecting sensitive personal information.
With these advantages, synthetic data is increasingly being adopted by tech companies. Research firm Gartner predicts it will become the primary form of data used in AI development by 2030.
Challenges of Relying on Synthetic Data
Despite its potential, synthetic data poses several challenges. One major concern is the risk of “model collapse,” where AI systems trained predominantly on synthetic data produce low-quality outputs riddled with errors, or “hallucinations.” For instance, AI models may struggle with spelling or semantic accuracy when trained on flawed synthetic datasets.
Another issue is the potential lack of nuance in synthetic data. Unlike real data, which reflects diverse scenarios and contexts, synthetic datasets can be overly simplistic, resulting in AI systems that lack depth and reliability.
Ensuring High-Quality Synthetic Data
To mitigate these issues, global standards for tracking and validating AI training data must be established. Organizations such as the International Organisation for Standardisation and the United Nations’ International Telecommunication Union could play a crucial role in implementing these systems worldwide.
AI systems should incorporate metadata tracking to trace the origins and quality of the synthetic data they use. Human oversight will also remain essential in defining objectives, validating data quality, and monitoring ethical compliance during training processes.
Additionally, AI algorithms can be leveraged to audit and verify synthetic datasets, ensuring consistency and accuracy by comparing them against real data benchmarks. This iterative process could enhance the quality of AI outputs and prevent systemic errors.
>>>BSB14G Replacement Battery for Ridgid R83015 130252003 130254002 130254008
The Path Forward
The future of AI hinges on maintaining high-quality data sources. While real data remains invaluable, synthetic data will play an increasingly prominent role in addressing shortages. When managed effectively, synthetic data could complement real data, enhancing AI systems’ accuracy, reliability, and ethical standards.
By adopting rigorous data validation practices and fostering global cooperation, the tech industry can ensure AI systems remain trustworthy and beneficial as they continue to evolve.