The field of weather forecasting has reached a significant milestone: researchers have introduced GenCast, an AI-driven weather prediction system developed by Google DeepMind. This system demonstrates faster and more accurate forecasts than the ENS model from the European Centre for Medium-Range Weather Forecasts (ECMWF), which has long been regarded as the global leader in weather prediction.
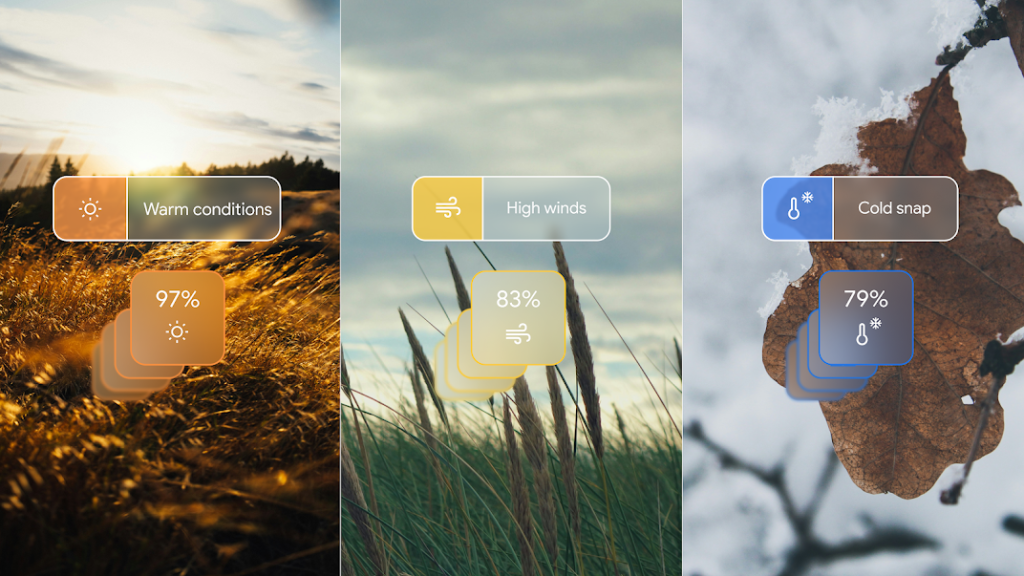
What are the advantages of GenCast?
Improved Accuracy
GenCast outperformed ENS by up to 20% in short-term weather forecasts and showed remarkable precision in predicting the paths of extreme weather events, such as hurricanes and cyclones, including their landfall locations.
Exceptional Efficiency
Unlike traditional physics-based models that require hours of computation on supercomputers, GenCast delivers results in just 8 minutes using a single Google Cloud TPU, a machine-learning-optimized processor.
Innovative Training
The model was trained on 40 years of historical weather data (1979–2018), encompassing a wide range of atmospheric variables such as wind speed, temperature, pressure, and humidity. GenCast builds on its predecessor, GraphCast, by producing probabilistic ensembles of 50 or more forecasts, offering greater reliability for predicting uncertain weather events.
Supportive Role
For now, GenCast is designed to complement rather than replace traditional physics-based methods, providing additional clarity for events such as heatwaves, cold spells, and high winds. Its applications could extend to sectors like renewable energy, where accurate forecasts help optimize power generation.
Implications for Weather Prediction
- Enhanced Ensemble Forecasting: GenCast’s ability to generate larger and more reliable ensembles provides improved confidence levels for extreme weather predictions.
- Reduced Computational Costs: The efficiency of GenCast makes high-resolution forecasts more accessible and reduces dependency on expensive computational resources.
- Transformative Potential: Experts, such as Sarah Dance from the University of Reading, have noted that this technology represents a paradigm shift in forecasting methodology, paving the way for broader adoption of AI-based approaches.
Challenges and Questions
While GenCast’s performance is promising, certain challenges remain. The authors have not answered whether their system has the physical realism to capture the ‘butterfly effect’, the cascade of fast-growing uncertainties, which is critical for effective ensemble forecasting.
The data GenCast trained on combines past observations with physics-based “hindcasts” that need sophisticated maths to fill gaps in historic data.
There is still a long way to go before machine learning approaches can completely replace physics-based forecasting.It remains to be seen whether generative machine learning can replace this step and go straight from the most recent unprocessed observations to a 15-day forecast.
The Road Ahead
GenCast is unlikely to replace traditional forecasting systems in the near future. Instead, it is expected to serve as a powerful assistive tool, augmenting current models and contributing to more accurate predictions. National weather services and industries reliant on precise weather information, such as energy and disaster management, are poised to benefit significantly.