Apple’s foray into artificial intelligence,Apple Intelligence, has been underwhelming, to say the least. The most glaring failure? Itsnews summaries, which faced widespread backlash for misreporting headlines and generatingfalse information. The issue became so severe that Applepaused the entire feature this weekuntil it can be fixed.
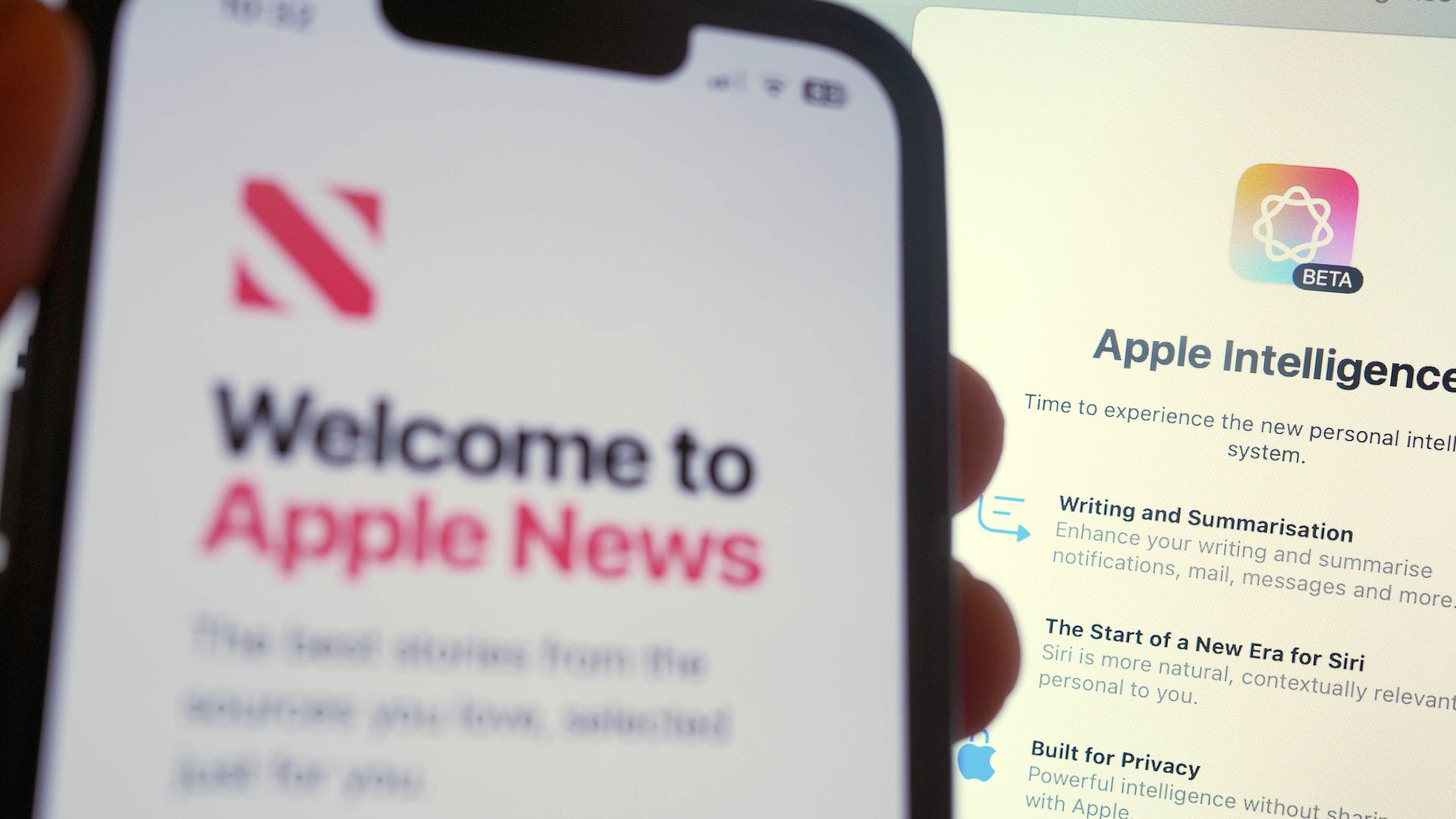
None of this should come as a surprise.AI “hallucinations”—instances where AI models generate incorrect or misleading information—are a well-documented issue with large language models (LLMs). To date, no one has found a true solution, and it’s unclear whether one even exists. But what makes Apple’s situation particularly reckless is thatits own engineers warned of these deficiencieswell before the company launched its AI system.
>>>S360X3BReplacement Battery forInsta360X3
Apple Knew AI Models Weren’t Ready
Last October, a group of Apple researchers published a study evaluating themathematical reasoningcapabilities of leading LLMs. The yet-to-be-peer-reviewed researchadded to the growing consensusthat AI models don’t actually “reason” in the human sense.
“Instead,”the researchers concluded,“they attempt to replicate the reasoning steps observed in their training data.”
In other words, these AI models aren’t truly thinking—they’re just mimicking patterns they’ve seen before.
Math Is Hard: How AI Fails at Simple Problems
To test AI reasoning, Apple’s researchers subjected 20 different models to thousands of math problems from the widely usedGSM8K dataset. These problems weren’t particularly difficult—most could be solved by a well-educated middle schooler. A typical question might read:
“James buys 5 packs of beef that are 4 pounds each. The price of beef is $5.50 per pound. How much did he pay?”
The key test came when researcherschanged the numbersin the problems to ensure the AI models weren’t just memorizing answers. Even this minor tweak caused a small butconsistent drop in accuracyacross all models.
But when the researchers went further—changing names and adding irrelevant details(such as mentioning that some fruits in a counting problem were “smaller than usual”)—the results were disastrous. Some models saw accuracy drop byas much as 65%.
Even thebest-performing model, OpenAI’s o1-preview, saw a17.5% decline, while its predecessor,GPT-4o, dropped by 32%. These results exposed a critical weakness: AI strugglesnot just with reasoning, but with identifying relevant informationfor problem-solving.
>>>U914479PHVReplacement Battery foriDataK3S
AI: More Copycat Than Thinker
The study’s conclusion was damning.”This reveals a critical flaw in the models’ ability to discern relevant information for problem-solving,” the researchers wrote. “Their reasoning is not formal in the common sense term and is mostly based on pattern matching.”
Put simply,AI models are great at appearing intelligent, and they often deliver the right answers—but only as long as they cancopy and repackagesolutions they’ve seen before. Once theycan’t rely on direct memorization, their performance crumbles.
This should have raised serious concerns about trusting an AI model tosummarize news—a process that involvesrearranging words while preserving meaning. Yet, Appleignored its own researchand pushed forward with Apple Intelligence anyway.
Then again, thistrial-and-error approachhas become standard practice across the AI industry. Apple’s misstep may be frustrating, but it’s hardly surprising.